本文已被:浏览 292次 下载 1312次
投稿时间:2022-04-01 修订日期:2022-09-26
投稿时间:2022-04-01 修订日期:2022-09-26
中文摘要: 高山滑雪运动对风极为敏感,风速尤其是极大风速往往是决定冬奥会赛事顺利进行的关键因素之一。利用2018—2021年1—3月欧洲中期天气预报中心(ECMWF)模式预报产品以及冬奥会延庆赛区8个关键点位的极大风速观测数据,基于决策树(DT)、随机森林(RF)和梯度提升决策树(GBDT)三种不同类型的机器学习算法分别构建极大风速客观预报模型。对比评估表明:极大风速预报的最佳预报因子主要集中在不同高度层的风速和风向,个别站还包括垂直速度,若去掉风向因子,绝大多数模型的预报准确率会降低,平均绝对误差会增加;总体上基于决策树基学习器集成学习的GBDT和RF模型要优于单一决策树模型DT,平均绝对误差最低的GBDT模型预报的极大风速误差介于1.56~3.57 m·s-1,较DT模型的改善率高达8.7%,同时,GBDT模型对超阈值极大风速的预报也较为优秀;随着预报点位海拔高度升高,各模型的平均绝对误差和准确率分别有增加和降低的趋势;随着预报时效增加,各模型的平均绝对误差表现出一定的周期性日变化特征。将表现优异的GBDT和RF两个模型作为初级学习器,以支持向量机模型作为次级学习器,基于stacking方法构建集成学习模型RGL。结果表明:集成模型对极大风速的预报较单一模型具备一定的改进能力,且以极大风速相对较大的高海拔站改进更为突出,平均绝对误差较单一模型最大可降低0.13 m·s-1,准确率最高可提升0.022。相关研究成果有力保障了2022年北京冬(残)奥会顺利进行。
中文关键词: 北京冬奥会,复杂地形,极大风,机器学习,集成学习
Abstract:Alpine skiing is extremely sensitive to wind, and especially extreme wind speed is often one of the key factors that determine the smooth progress of the Winter Olympic Games. The numerical model data from the European Center for Medium-Range Weather Forecasts (ECMWF) and corresponding extreme wind observations at eight key stations in Yanqing Competition Zone of the Winter Olympic Games from January to March during 2018-2021 are used. The objective forecasting models of extreme wind speed are constructed based on three types of machine learning algorithms: decision tree (DT), random forest (RF) and gradient boosting decision tree (GBDT). The comparative evaluation results show that the best predictors of extreme wind speed mainly focus on the wind speed and direction at different levels, and additionally include the vertical velocity at individual stations. Removing the wind direction leads to the decrease of accuracy and increase of mean absolute error (MAE) in most of models. On the whole, the GBDT and RF models based on the decision tree ensemble learning are superior to the single decision tree model (DT). The GBDT model has the least MAE ranging from 1.56 m·s-1 to 3.57 m·s-1, and the maximum improvement rate is up to 8.7% compared with the DT model. Besides, the GBDT model is also skillful in the forecasts of super threshold extreme wind speed. All models have the increasing trend in the MAE and decreasing trend in the accuracy with the rising elevation of stations. As the forecasting lead time extends, the MAE of each model shows a periodic diurnal variation. Based on the stacking ensemble learning method, the RGL model is established using the two outstanding models, GBDT and RF, as the primary learner and the support vector machine as the secondary learner. The results indicate that compared with the single model, the RGL model has a certain ability to improve the prediction of extreme wind speed, especially at the high-altitude stations with relatively high winds. The MAE can be reduced by a maximum of 0.13 m·s-1, and the accuracy can be increased by a maximum of 0.022. The relevant research results have been well applied to the 2022 Beijing Winter Olympic and Paralympic Games.
keywords: Beijing Winter Olympic Games, complex terrain, extreme wind, machine learning, ensemble learning
文章编号: 中图分类号: 文献标志码:
基金项目:国家重点研发计划(2018YFF0300104)、北京市科技计划(Z201100005820002)和山西省气象局重点项目(SXKZDTQ20236301)共同资助
作者 | 单位 |
邱贵强 | 山西省气象台,太原 030006 |
于波 | 北京市气象台,北京 100089 |
陶亦为 | 国家气象中心,北京 100081 |
阎宏亮 | 北京市延庆区气象局,北京 102100 |
王扬 | 山西省气象台,太原 030006 |
引用文本:
邱贵强,于波,陶亦为,阎宏亮,王扬,2023.基于集成学习算法的冬奥会延庆赛区极大风速预报[J].气象,49(6):721-732.
QIU Guiqiang,YU Bo,TAO Yiwei,YAN Hongliang,WANG Yang,2023.Forecasting of Extreme Wind Speed in Yanqing Competition Zone of the Winter Olympic Games Based on Ensemble Learning Algorithm[J].Meteor Mon,49(6):721-732.
邱贵强,于波,陶亦为,阎宏亮,王扬,2023.基于集成学习算法的冬奥会延庆赛区极大风速预报[J].气象,49(6):721-732.
QIU Guiqiang,YU Bo,TAO Yiwei,YAN Hongliang,WANG Yang,2023.Forecasting of Extreme Wind Speed in Yanqing Competition Zone of the Winter Olympic Games Based on Ensemble Learning Algorithm[J].Meteor Mon,49(6):721-732.
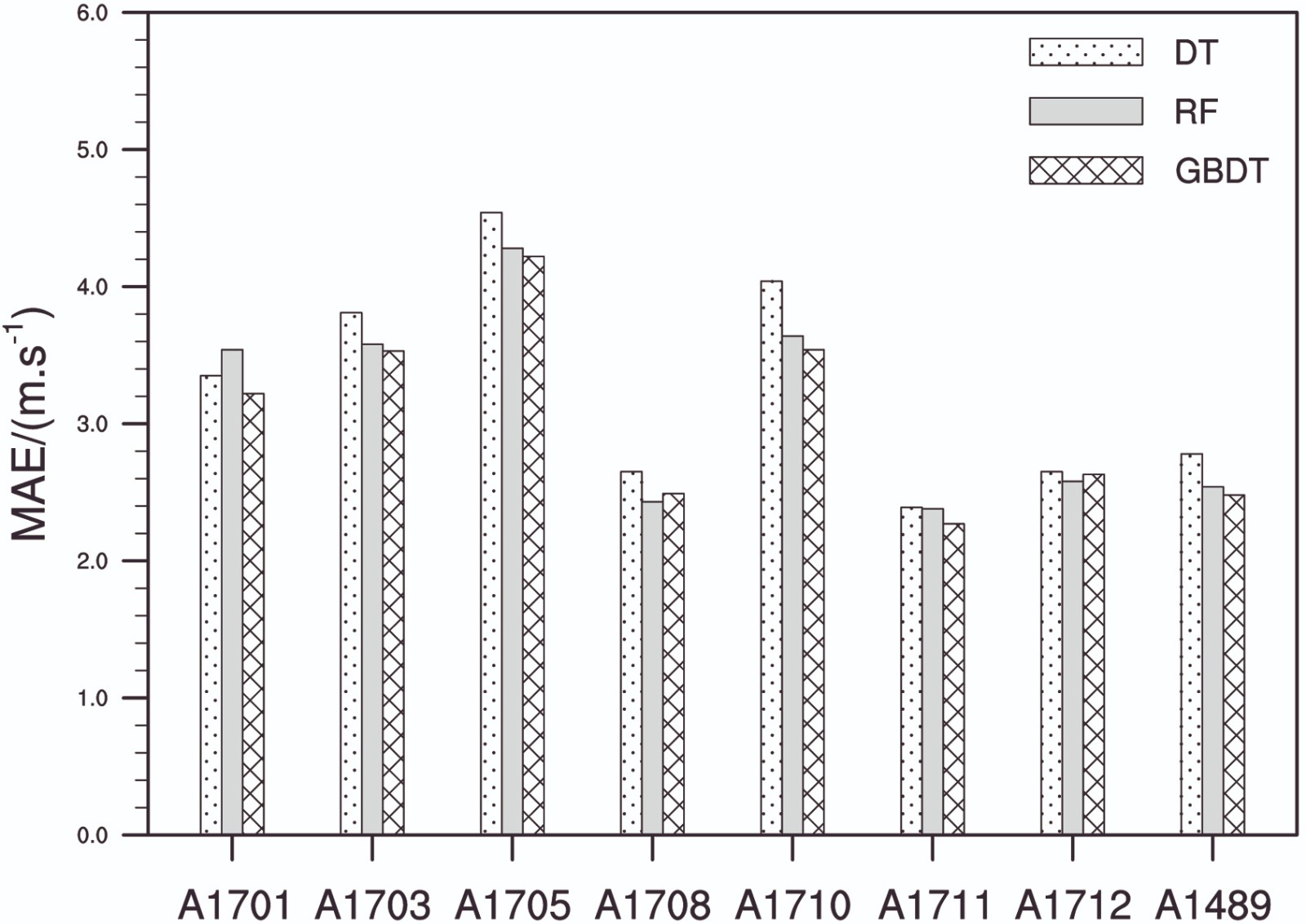