本文已被:浏览 377次 下载 2215次
投稿时间:2021-08-04 修订日期:2022-07-01
投稿时间:2021-08-04 修订日期:2022-07-01
中文摘要: 基于2017—2019年欧洲中期天气预报中心的全球预报系统(ECMWF-IFS),结合对应时间段站点观测实况,采用深度学习方法建立了多层全连接神经网络模型(简称DL模型),对未来84 h的地面气温进行订正预报。使用湖南省2020年全年的预报结果进行对比检验评估,得到以下结论:从空间分布来看,DL模型平均均方根误差(RMSE)在大部分地区为1.5~2.0℃(全省平均RMSE为1.78℃),其对ECMWF-IFS模式的订正效果明显,尤其是在高海拔地区,改善率随着预报站点海拔的增加而上升;数值预报的RMSE有明显的日变化特征,每日午后误差最大,DL模型的改善幅度也最大,日出前时效误差最小,改善率在不同海拔高度有不同的日变化特征;DL模型每个月相对ECMWF-IFS的平均RMSE都有明显的订正效果,其中10月、11月RMSE改善率最高,12月最低;从2020年年底一次寒潮过程的日最高、最低及逐3 h气温检验效果来看,DL模型对ECMWF-IFS的系统误差有明显的订正效果,在单站的曲线上,也能看出DL模型更接近观测实况。评估结果表明:模型可以显著地减小ECMWF-IFS的预报误差,其输出结果基本满足日常预报业务的需求。
中文关键词: 气温预报,深度学习,应用与评估
Abstract:By using ECMWF-IFS model data and observation data, this study established a fully connected neural network model (DL), tried to make more accurate temperature forecast based on deep learning method for the future 84 hours. The comparative evaluation of ECMWF-IFS and DL was made in 2020. The results show that DL correction can improve the ECMWF-IFS forecasts. The root mean square error (RMSE) of DL in most areas of Hunan is 1.5-2.0℃. There is a significant correction effect for ECMWF-IFS, and the improvement rate increases with the altitude of stations. The diurnal variation is also obvious for the RMSE of ECMWF-IFS/DL model. The RMSE is higher in the afternoon (with higher improvement rate), but lower before sunrise. The improvement rate has different diurnal variation characteristics at different altitudes. DL model shows higher accuracy in the whole year, of which the higher improvement rate appears in October and November (lower in December). In addition, the forecast results in a cold wave process was evaluated. In the accuracy for daily maximum/minimum temperature and the RMSE for 3 h temperature, DL model shows obvious correction ability for the systematic deviation from ECMWF-IFS. The DL temperature curve for single station is much closer to observation than the ECMWF-IFS forecast. Thus, the model can significantly reduce numerical weather prediction error, and its products could basically meet the demands of daily forecast service.
文章编号: 中图分类号:P456,P457 文献标志码:
基金项目:湖南省气象局第三期业务能力建设项目(NLJS01)、湖南省气象局重点项目(XQKJ17A004)共同资助
引用文本:
陈鹤,蔡荣辉,陈静静,傅承浩,周莉,陈龙,2022.基于深度学习方法的气温预报技术应用与评估[J].气象,48(11):1373-1383.
CHEN He,CAI Ronghui,CHEN Jingjing,FU Chenghao,ZHOU Li,CHEN Long,2022.Application and Evaluation of Temperature Forecast Based on Deep Learning Method[J].Meteor Mon,48(11):1373-1383.
陈鹤,蔡荣辉,陈静静,傅承浩,周莉,陈龙,2022.基于深度学习方法的气温预报技术应用与评估[J].气象,48(11):1373-1383.
CHEN He,CAI Ronghui,CHEN Jingjing,FU Chenghao,ZHOU Li,CHEN Long,2022.Application and Evaluation of Temperature Forecast Based on Deep Learning Method[J].Meteor Mon,48(11):1373-1383.
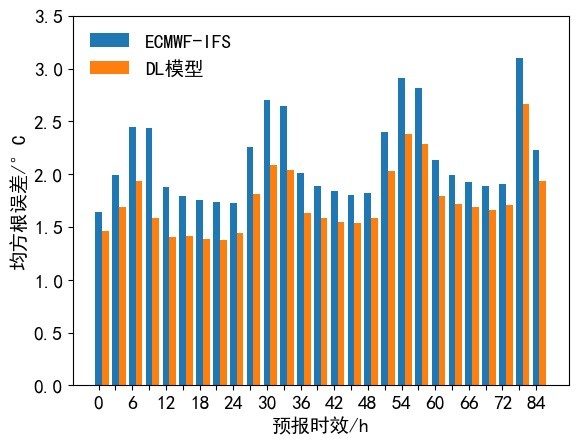