本文已被:浏览 1次 下载 0次
投稿时间:2024-01-15 修订日期:2025-01-08
投稿时间:2024-01-15 修订日期:2025-01-08
中文摘要: 为了提高降水临近预报的准确性,提出一种深度神经网络相结合的对抗神经网络模型CastNet。该模型借助循环神经网络捕捉雷达回波数据的时空特征,运用对抗神经网络模拟云团生消变化,再将光流约束融入神经网络引导模型训练,加速神经网络的学习过程并增强模型的时空一致性,有效地解决了预报模糊性问题,提升降水强度与位置的准确性。对2023年5—10月广西及周边区域的9次主要降水过程进行检验,结果表明:在不同降水强度(≥0.1、≥2、≥7、≥15、≥25、≥40 mm·h-1)下,SWAN 2.0的平均TS评分分别为0.458、0.270、0.085、0.034、0.014和0.003;SWAN 3.0的平均TS评分分别为0.452、0.402、0.225、0.129、0.085和0.048;CastNet模型的平均TS评分分别为0.439、0.397、0.225、0.139、0.104和0.073。即:除个别持平外,CastNet在≥7 mm·h-1及以上高降水强度中评分高于SWAN 2.0和SWAN 3.0。此外,随着预报时效延长,CastNet的相对优势更为明显。
Abstract:To improve the accuracy of precipitation nowcasting, this paper proposes an adversarial neural network model named CastNet that combines deep neural networks. This model utilizes the recurrent neur-al network to capture the spatio-temporal features of radar echo data, employs the adversarial neural network to simulate the generation and dissipation changes of cloud clusters, and then integrates the optical flow constraint into the neural network to guide the model training. This accelerates the learning process of the neural network and enhances the spatio-temporal consistency of the model, effectively solving the problem of forecast ambiguity and significantly improving the accuracy of precipitation intensity and location. Tests are conducted on 9 major precipitation processes in Guangxi and its surrounding areas from May to October 2023. The results show that under various precipita-tion intensities (≥0.1, ≥2, ≥7, ≥15, ≥25, ≥40 mm·h-1), the average TS scores of SWAN 2.0 are 0.458, 0.270, 0.085, 0.034, 0.014 and 0.003, respectively; the average TS scores of SWAN 3.0 are 0.452, 0.402, 0.225, 0.129, 0.085 and 0.048, respectively; and the average TS scores of the CastNet model are 0.439, 0.397, 0.225, 0.139, 0.104 and 0.073, respectively. It can be seen clearly that the TS scores by the CastNet are higher than those of SWAN 2.0 and SWAN 3.0 under high-intensity precipita-tion of ≥7 mm·h-1 and above, except for few data points that are flat. In addition, as the forecast lead time extends, the relative advantage of CastNet becomes more obvious.
文章编号: 中图分类号: 文献标志码:
基金项目:广西智能网格预报服务创新团队专项、广西自然科学基金项目(2022GXNSFAA035482)和广西气象科研计划指令性项目(桂气科ZL01)共同资助
引用文本:
曾小团,谭肇,沈玉伟,范娇,黄荣成,周弘媛,梁潇,黄大剑,2025.CastNet:深度学习定量降水临近预报模型[J].气象,51(4):400-416.
ZENG Xiaotuan,TAN Zhao,SHEN Yuwei,FAN Jiao,HUANG Rongcheng,ZHOU Hongyuan,LIANG Xiao,HUANG Dajian,2025.CastNet: Deep-Learning-Based Model for Quantitative Precipitation Nowcasting[J].Meteor Mon,51(4):400-416.
曾小团,谭肇,沈玉伟,范娇,黄荣成,周弘媛,梁潇,黄大剑,2025.CastNet:深度学习定量降水临近预报模型[J].气象,51(4):400-416.
ZENG Xiaotuan,TAN Zhao,SHEN Yuwei,FAN Jiao,HUANG Rongcheng,ZHOU Hongyuan,LIANG Xiao,HUANG Dajian,2025.CastNet: Deep-Learning-Based Model for Quantitative Precipitation Nowcasting[J].Meteor Mon,51(4):400-416.
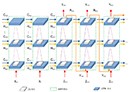