本文已被:浏览 1次 下载 1次
投稿时间:2024-08-23 修订日期:2025-01-03
投稿时间:2024-08-23 修订日期:2025-01-03
中文摘要: 根据飞鸟回波在天气雷达反射率产品上呈现明显的圆环形态这一具体图像特征,提出一种基于轻量化卷积神经网络(YOLOv5)与多目标跟踪算法(DeepSort)相结合的改进算法,利用2020—2023年营口雷达体扫回波强度资料,构造模型训练数据集和测试数据集,分别对鸟回波进行识别和追踪。首先,在YOLOv5算法中引入轻量级注意力机制以提高整体模型检测的准确性与有效性;其次,在DeepSort算法中将原有的交并比IOU匹配机制替换为一种改进的目标检测的损失函数DIOU匹配机制,DIOU在计算边界框重叠度的基础上,引入了边界框中心点之间的距离,从而提供更精确的定位,减少了因部分遮挡重叠等原因造成的追踪目标编号ID错误匹配和ID切换次数。试验结果表明,优化后的YOLOv5算法在精准度方面提升了2.6百分点,召回率提升了1.0百分点,阈值大于0.5的平均精准度提升了1.2百分点;改进后的DeepSort算法使得ID切换次数降低2次,多目标跟踪准确率提高了4.5百分点,实现对初始模型的轻量化;整体检测性能得到明显提高,满足对鸟回波识别与追踪的实际需求。
中文关键词: 深度学习,注意力机制,目标检测,目标追踪
Abstract:According to the specific image feature that the bird echo shows obvious ring shape in the weather radar reflectivity product, this article proposes an improved algorithm based on a lightweight convolutional neural network You Only Look Once Version5 (YOLOv5) and multi-object tracking based on deep learning based simple online and realtime tracking (DeepSort). The training and test datasets are constructed based on radar volume scanning echo intensity. Data obtained from the Yingkou Weather Radar from 2020 to 2023. The bird echoes are tracked, respectively. Firstly, Shuffle Attention (SA), a lightweight attention mechanism, is introduced into YOLOv5 algorithm to improve the accuracy and effectiveness of the overall model checking. Secondly, in DeepSort algorithm, the original cross-merge-ratio intersection over union (IOU) matching mechanism is replaced by an improved loss function of object detection, distance-intersection over union (DIOU) matching mechanism. DIOU introduces the distance between the center points of the boundary box on the basis of calculating the overlap degree of the boundary box, so as to provide more accurate positioning. The number of identification (ID) error matching and ID switching caused by partial occlusion overlap is reduced. The test results show that the optimized YOLOv5 algorithm improves the accuracy by 2.6 percentage point, the recall rate by 1 percentage point, and the average accuracy of threshold values greater than 0.5 by 1.2 percentage point. The improved DeepSort algorithm reduces the number of ID switches by 2 times, and multi target tracking accuracy multi-object tracking accuracy (MOTA) increases by 4.5 percentage point, thus improves lightweight of the initial model. Generally, the overall checking performance is significantly improved, and may meet the actual demand for bird echo recognition and tracking.
文章编号: 中图分类号: 文献标志码:
基金项目:四川省科技厅重点研发项目(2023YFG0170)、中国气象局沈阳大气环境研究所和东北冷涡重点开放实验室联合开放基金项目(2023SYIAEKFMS08)和中国气象科学研究院基本科研业务费(2023Z019)共同资助
作者 | 单位 |
姚文 | 中国气象局沈阳大气环境研究所,沈阳 110166;辽宁省营口市气象局,营口 115001 |
李松书 | 成都信息工程大学,成都 610621 |
王海江 | 成都信息工程大学,成都 610621 |
张晶 | 中国气象局沈阳大气环境研究所,沈阳 110166;辽宁省营口市气象局,营口 115001 |
引用文本:
姚文,李松书,王海江,张晶,2025.基于YOLOv5与DeepSort对天气雷达数据鸟杂波的识别与追踪[J].气象,51(4):417-430.
YAO Wen,LI Songshu,WANG Haijiang,ZHANG Jing,2025.Identification and Tracking of Bird Clutter in Weather Radar Data Based on YOLOv5 and DeepSort[J].Meteor Mon,51(4):417-430.
姚文,李松书,王海江,张晶,2025.基于YOLOv5与DeepSort对天气雷达数据鸟杂波的识别与追踪[J].气象,51(4):417-430.
YAO Wen,LI Songshu,WANG Haijiang,ZHANG Jing,2025.Identification and Tracking of Bird Clutter in Weather Radar Data Based on YOLOv5 and DeepSort[J].Meteor Mon,51(4):417-430.
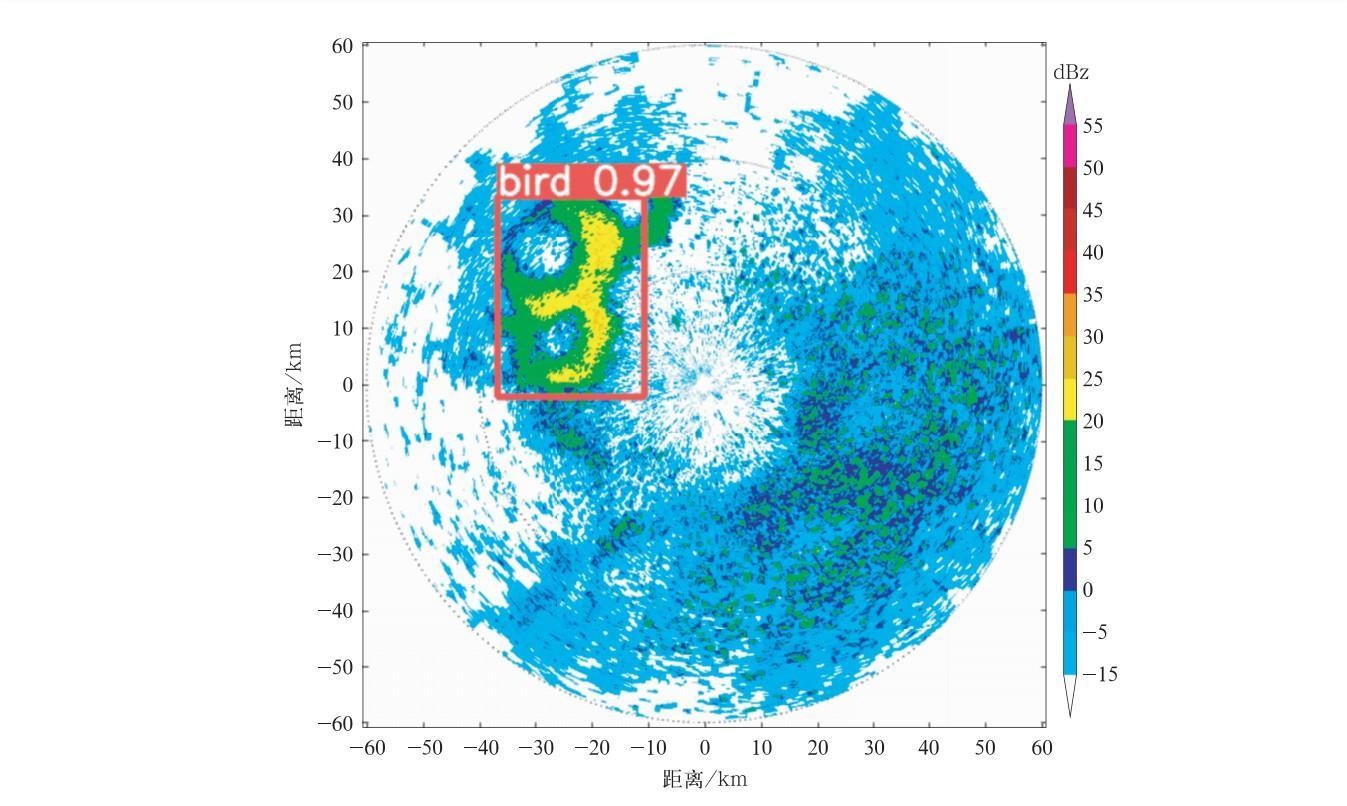