本文已被:浏览 123次 下载 890次
投稿时间:2023-06-05 修订日期:2024-03-28
投稿时间:2023-06-05 修订日期:2024-03-28
中文摘要: 针对传统方法在捕捉气象序列长期依赖关系及泛化性能上的不足,提出了一种基于稀疏注意力与自适应时序分解的气温预报模型(ATFSAS)。该模型整体采用编码器 解码器架构,结合稀疏注意力机制以有效捕捉气象观测数据间的长期依赖性。为减少编码过程中造成的冗余,提出了一种信息蒸馏方法。通过结合多层解码器与自适应时序分解单元,逐步细化预报信号中的周期性和趋势性分量,实现了较为精准的气温预报。基于德国耶拿气象数据集,进行24 h精细化气温预报,其平均绝对误差为1.7108℃。基于中国地面气候资料日值数据集,进行中短期日平均气温预报和多地区单日平均气温预报,相比传统模型LSTM,ATFSAS模型预报结果的预报性能分别提升了35.56%和23.66%。
中文关键词: 人工智能,深度学习,时序预测,气温预报
Abstract:Addressing the limitations of traditional models in capturing long-term dependencies and generalization ability in meteorological sequences, we propose a novel air temperature forecasting model based on sparse attention and adaptive seasonal and trend decomposition using loess (ATFSAS) in this paper. ATFSAS employs an encoder-decoder architecture, and integrates a sparse attention mechanism to effectively capture long-term dependencies in meteorological observation data. An information distillation method is introduced to reduce redundancy during the encoding process. The model refines the periodic and trend components in the forecast signals by combining a multi-layer decoder with an adaptive timing decomposition unit, achieving precise air temperature forecast. Based on the climate dataset in Jena, Germany, 24 h refined air temperature forecast is performed by ATFSAS, abtaining a mean absolute error of 1.7108℃. Compared to the LSTM model, ATFSAS demonstrates superior performance in medium-short term daily average air temperature and multi-region single-day average air temperature forecasts based on the China ground climate daily dataset, and their mean absolute errors get improved by 35.56% and 23.66%, respectively.
文章编号: 中图分类号: 文献标志码:
基金项目:国家重点研发计划(2021YFE0104400)和国家自然科学基金项目(62372243、62102187、42175194)共同资助
引用文本:
杨彬,马廷淮,黄学坚,2024.基于注意力机制与自适应时序分解的气温预报模型[J].气象,50(6):723-732.
YANG Bin,MA Tinghuai,HUANG Xuejian,2024.Air Temperature Forecasting Model Based on Attention Mechanism and AdaSTL[J].Meteor Mon,50(6):723-732.
杨彬,马廷淮,黄学坚,2024.基于注意力机制与自适应时序分解的气温预报模型[J].气象,50(6):723-732.
YANG Bin,MA Tinghuai,HUANG Xuejian,2024.Air Temperature Forecasting Model Based on Attention Mechanism and AdaSTL[J].Meteor Mon,50(6):723-732.
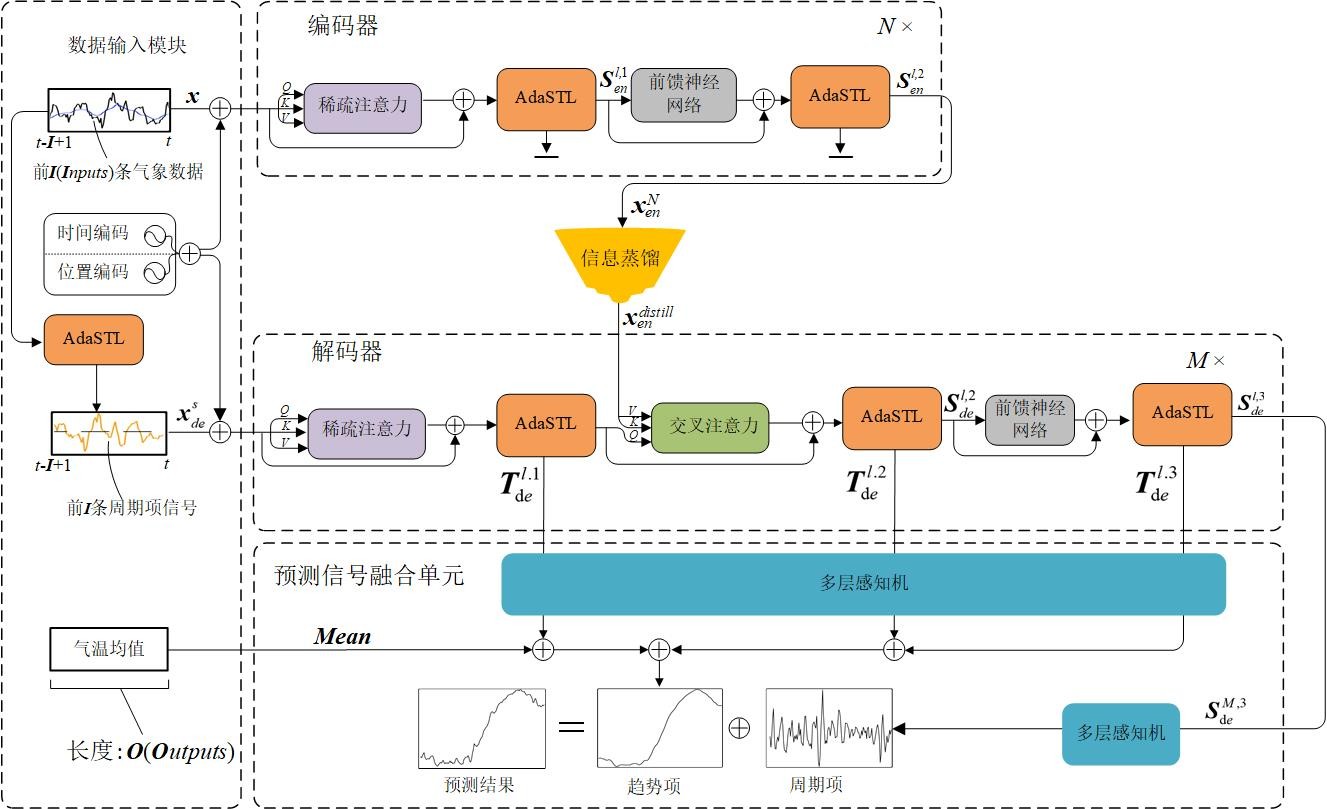