本文已被:浏览 33次 下载 546次
投稿时间:2024-12-03 修订日期:2025-03-13
投稿时间:2024-12-03 修订日期:2025-03-13
中文摘要: 洪水预报是降低洪灾损失、提升防灾减灾能力非工程措施的有效途径,实现精准洪水预报是水文领域的关键技术挑战之一。目前,基于物理机制的洪水预报模型在模拟精度和效率上仍有不足,而采用深度学习技术构建的预报模型则得到了迅猛发展。文章全面回顾和总结了洪水预报领域所应用的深度学习模型的原理和特点,及其在洪水定量和概率预报中的应用进展和存在问题。聚焦介绍和探讨了深度学习模型与洪水物理模型在物理过程参数化、可解释性研究、洪水预报模型误差校正等方面的契合点和应用前景。分析认为,深度学习未来将走向与物理模型的深度耦合,成为洪水时间序列预报的重要发展范式,并将是实现未来水利智慧化的重要研究内容。最后针对深度学习在洪水预报中的难点给出几点思考,对当前面临的挑战提出几点相应的解决方案,以便更好地在洪水预报领域探索应用深度学习技术。
中文关键词: 深度学习,洪水预报,定量预报,概率预报,耦合物理模型
Abstract:Flood forecasting is an effective non engineering measure to reduce the economic losses brought by floods. Accurately forecasting flood is one of the key technical challenges in hydrological field. There are flood forecasting models based on physical mechanisms used, but the accuracy and efficiency of forecasts need to be improved. At present, forecasting models constructed by using deep learning techniques have been developed rapidly. This article comprehensively reviews the principles and characteristics of deep learning models that have been applied in the field of flood forecasting and summarizes their application progresses and problems in the quantitative and probabilistic flood forecasting. In addition, this article explores the relevance and application prospects of deep learning models combined with flood physics models, particularly in the parameterization of physical processes, interpretability studies, and error correction of flood forecasting models. The results suggest that the deep coupling of deep learning technology with physical models is the developing direction of deep learning models in the future. It will be an important development paradigm for the time series prediction of flood, and also an important research component to achieve intelligent water resource management in the future. Finally, to better apply the deep learning technology in the field of flood forecasting, some thoughts on the difficulties of deep learning in flood prediction are given and corresponding solutions are proposed for the current challenging problems.
文章编号: 中图分类号: 文献标志码:
基金项目:湖北省自然科学气象创新发展联合基金项目(2022CFD129、2023AFD094)、中国气象局创新发展专项(CXFZ2024P043)、长江流域气象开放基金项目(CJLY2022Y06)、湖北省气象局面上基金项目(2022Y06)、贵州省科技厅自然科学面上项目(黔科合基础 zk[2025]面上319)、湖南省气象局重点项目(XQKJ22A005)、中国气象局流域强降水重点开放实验室基金项目(2023BHR Y26)和中国气象局武汉暴雨研究所基本科研业务专项(WHIHRKYYW202404)共同资助
引用文本:
祁海霞,彭涛,智协飞,季焱,殷志远,沈铁元,王俊超,向怡衡,胡泊,2025.深度学习技术在洪水预报中的应用进展及思考[J].气象,51(4):446-459.
QI Haixia,PENG Tao,ZHI Xiefei,JI Yan,YIN Zhiyuan,SHEN Tieyuan,WANG Junchao,XIANG Yiheng,HU Po,2025.Progress and Reflection on Application of Deep Learning Techniques in Flood Forecasting[J].Meteor Mon,51(4):446-459.
祁海霞,彭涛,智协飞,季焱,殷志远,沈铁元,王俊超,向怡衡,胡泊,2025.深度学习技术在洪水预报中的应用进展及思考[J].气象,51(4):446-459.
QI Haixia,PENG Tao,ZHI Xiefei,JI Yan,YIN Zhiyuan,SHEN Tieyuan,WANG Junchao,XIANG Yiheng,HU Po,2025.Progress and Reflection on Application of Deep Learning Techniques in Flood Forecasting[J].Meteor Mon,51(4):446-459.
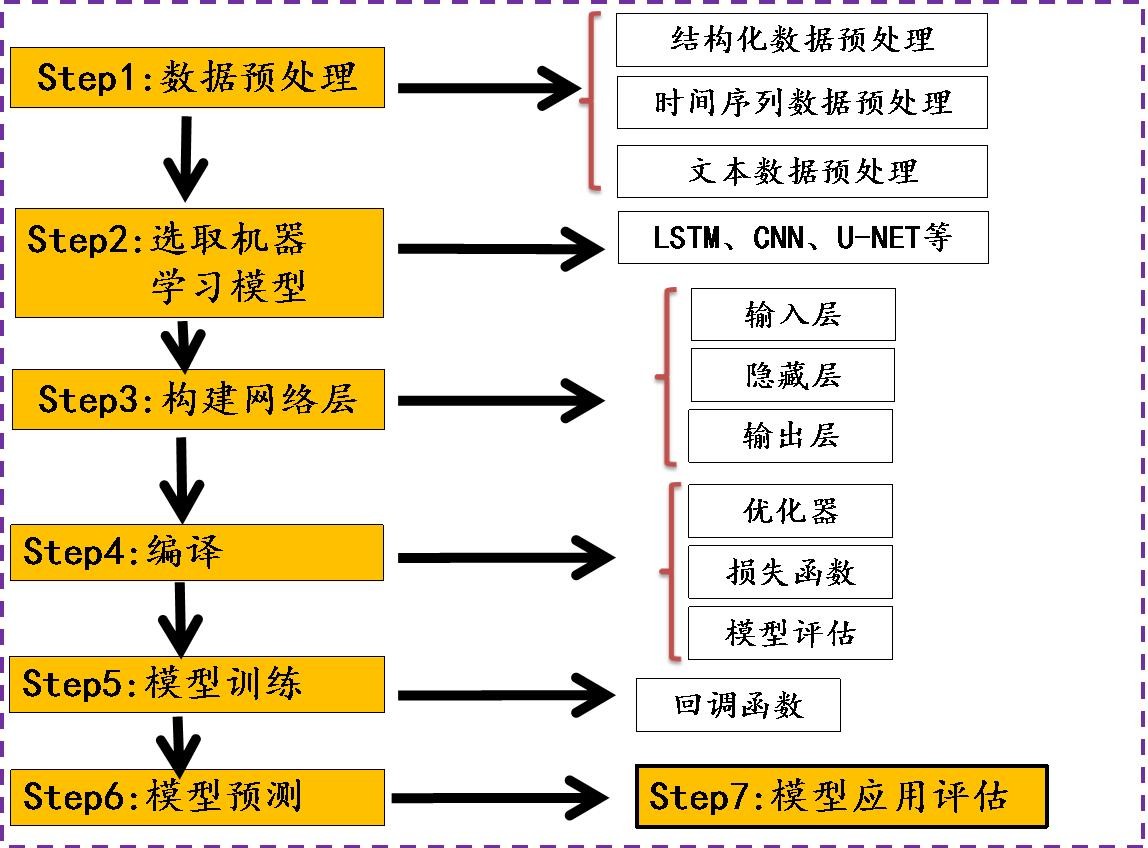