本文已被:浏览 966次 下载 2754次
投稿时间:2018-03-11 修订日期:2019-06-10
投稿时间:2018-03-11 修订日期:2019-06-10
中文摘要: 数值模式的直接输出预报在实际应用时常与实况产生一定的偏差,对模式预报进行有效的本地化订正是提高预报准确率的重要手段。以欧洲中期天气预报中心(ECMWF)模式细网格资料,采用最优集合(anolog ensemble,AnEn)预报订正方法对北京市各站1~7 d的日最高气温和日最低气温进行订正,并对相关参数进行了本地化。采用了滑动训练期、优化变量权重两种方案进行训练。检验评估结果表明:(1)滑动训练期采用60 d时能同时保证计算效率和预报准确率;采用最优变量权重方案后,与预报员主观预报准确率对比,AnEn的最低气温优于预报员主观预报,最高气温基本相当;增加训练期的长度(引入多年的历史资料)相比优化变量权重方案能更有效地提高预报准确率。(2)AnEn预报订正方法在改善数值模式预报的固有偏差(如对由数值模式对局地地形、边界层日变化等形成的误差)效果显著,有较好的应用价值;对于因局地天气(如霾、降水、大风等)影响下,AnEn的温度预报准确率虽优于ECMWF,但不如主观预报,未来还有改进空间。还对检验结果进行了时间和空间验证,确保在以后的业务尤其是智能网格预报业务中的运行效果。
中文关键词: 最优集合,客观温度预报,滑动训练期,优化变量权重
Abstract:Model-based numerical prediction is often affected by bias when compared to local observations. In this study, the European Center for Medium-Range Weather Forecasting (ECMWF) data were used to generate the analog ensemble (AnEn) prediction over the 15 national weather stations and 274 automatic stations of Beijing, with a focus on correcting ECMWF prediction of the daily maximum and minimum temperatures, 1-7 day ahead, twice a day. The analog of a forecast for a given location and time is defined as the observation that corresponds to a past prediction matching selected features of the current forecast. The best analogs form AnEn, which produces accurate predictions and a reliable quantification of their uncertainty with similar or superior skill compared to traditional ensemble methods while requiring considerably less real-time computational resources. An analysis of the performance of ECMWF and AnEn in space and time was presented. The results demonstrate that a short training period of 60 days may be a good compromise for the computational efficiency and the quality of deterministic predictions. Extending the training periods would further increase the prediction quality than optimizing the environmental parameters, no matter 1-month, 3-month or 6-month optimizations. AnEn correction results are better than the predictions generated by the forecasters, particularly for daily minimum temperatures. AnEn effectively reduces the bias of ECMWF predictions, resulting in a skilled downscaled prediction at the observation location, consistently over time and space. However, AnEn is not very effective in improving predictions of haze, precipitation, and strong winds, which may require a much longer training data set. Furthermore, this study tests the results over time and space to make sure the method’s reliability for the future smart grid forecast operation.
文章编号: 中图分类号: 文献标志码:
基金项目:国家重点研发计划(2018YFC1507305和2018YFF0300104)、中央级公益性科研院所基本科研业务费专项(IUMKY201719)和中国气象局发展预报业务检验评估关键技术项目[YBGJXM(2017)06-4]共同资助
作者 | 单位 |
郝翠 | 北京城市气象研究院,北京 100089 北京市气象台,北京 100089 |
张迎新 | 北京市气象台,北京 100089 |
王在文 | 北京城市气象研究院,北京 100089 |
付宗钰 | 北京市气象台,北京 100089 |
DELLE MONACHE Luca | National Center for Atmosphereic Research, Boulder, USA |
引用文本:
郝翠,张迎新,王在文,付宗钰,DELLE MONACHE Luca,2019.最优集合预报订正方法在客观温度预报中的应用[J].气象,45(8):1085-1092.
HAO Cui,ZHANG Yingxin,WANG Zaiwen,FU Zongyu,DELLE MONACHE Luca,2019.Application of Analog Ensemble Rectifying Method in Objective Temperature Prediction[J].Meteor Mon,45(8):1085-1092.
郝翠,张迎新,王在文,付宗钰,DELLE MONACHE Luca,2019.最优集合预报订正方法在客观温度预报中的应用[J].气象,45(8):1085-1092.
HAO Cui,ZHANG Yingxin,WANG Zaiwen,FU Zongyu,DELLE MONACHE Luca,2019.Application of Analog Ensemble Rectifying Method in Objective Temperature Prediction[J].Meteor Mon,45(8):1085-1092.
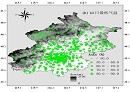