本文已被:浏览 82次 下载 343次
投稿时间:2022-06-21 修订日期:2023-07-30
投稿时间:2022-06-21 修订日期:2023-07-30
中文摘要: 为了提高短时强降水预报准确性,在2019—2020年4—9月福建省逐时降水实况观测资料与中国气象局广东快速更新同化数值预报系统(CMA-GD)模式预报产品的基础上,应用LightGBM集成学习算法框架,建立以30 mm·h-1为阈值的逐时降水预报模型。通过特征处理、自助聚合及超参数搜索等技术对模型进行优化,结合AUC、AUPR与传统分类指标,设计了包括业务模拟测试在内的多项试验,通过对比各建模方案验证了模型对于较长时效的短时强降水预报的适用性。结果表明:模式预报本身的命中率和空报率均较高,各建模方案具有不同程度的改善作用。自助聚合可以增强模型预测稳定性,轻微不平衡子训练集能降低模型预测空报率而取得更高的综合评分,在验证集中最佳TS评分可达17.5%;对分类信息增益贡献最大的特征变量为K指数,其次为500 hPa露点温度和时间参数特征;试验指标从优到劣依次为:随机交叉验证、小时划分的随机交叉验证、业务模拟测试,可见模型有效性主要来自相同或相邻时刻的样本信息;设计基于逻辑回归的异质模型动态融合方案以改善静态同质模型表现,各项指标均有小幅提升,在命中率接近50%时削减空报样本超过52万个。
中文关键词: LightGBM,短时强降水预报,样本不平衡,动态融合
Abstract:In order to improve the accuracy of short-time severe rainfall forecasts, the LightGBM algorithm is applied to build the hourly precipitation forecasting model based on the precipitation observation data and CMA-GD model forecast products of Fujian Province from April to September in 2019 and 2020. Correction models are optimized by the feature processing, Bagging (bootstrap aggregating) and hyperparameter searching. Combined with AUC, AUPR and traditional classification indices, a series of experiments are designed to evaluate different modeling schemes and verify the applicability in short-time severe rainfall forecasting. The results show that all modeling schemes can improve the original numerical model forecast representing the high POD and FAR in varying degrees. Bagging can enhance the stability of model prediction, and the slightly unbalanced sub-training set contributes to the higher TS scores by reducing the FAR with the best TS score of validation set being about 17.5%. The largest contribution of feature variable to the classification information gain is K index, followed by 500 hPa dew point and time parameters. The ranking of experiment indices in good to bad order is random cross-validation, random hourly cross-validation and operational simulation test which indicates that the validity of correction models mainly result from the sample information at the same or adjacent moments. The dynamic fusion scheme of heterogeneous models based on logistic regression increases indices of static homogeneous models, which decreases at least 520 〖KG-*5〗000 false alarm samples with approximately 50% POD.
文章编号: 中图分类号: 文献标志码:
基金项目:厦门市气象局科技专项(3502Z20214ZD4014)、中国气象局复盘总结专项(FPZJ2023-065)和中国气象局/广东省区域数值天气预报重点实验室开放基金课题(J202005)共同资助
引用文本:
陈锦鹏,黄奕丹,朱婧,林辉,程晶晶,杨德南,2024.集成学习和动态融合算法在福建省短时强降水预报中的应用[J].气象,50(1):48-58.
CHEN Jinpeng,HUANG Yidan,ZHU Jing,LIN Hui,CHENG Jingjing,YANG Denan,2024.Application of Ensemble Learning and Dynamic Fusion for Short-Time Severe Rainfall Forecasting in Fujian Province[J].Meteor Mon,50(1):48-58.
陈锦鹏,黄奕丹,朱婧,林辉,程晶晶,杨德南,2024.集成学习和动态融合算法在福建省短时强降水预报中的应用[J].气象,50(1):48-58.
CHEN Jinpeng,HUANG Yidan,ZHU Jing,LIN Hui,CHENG Jingjing,YANG Denan,2024.Application of Ensemble Learning and Dynamic Fusion for Short-Time Severe Rainfall Forecasting in Fujian Province[J].Meteor Mon,50(1):48-58.
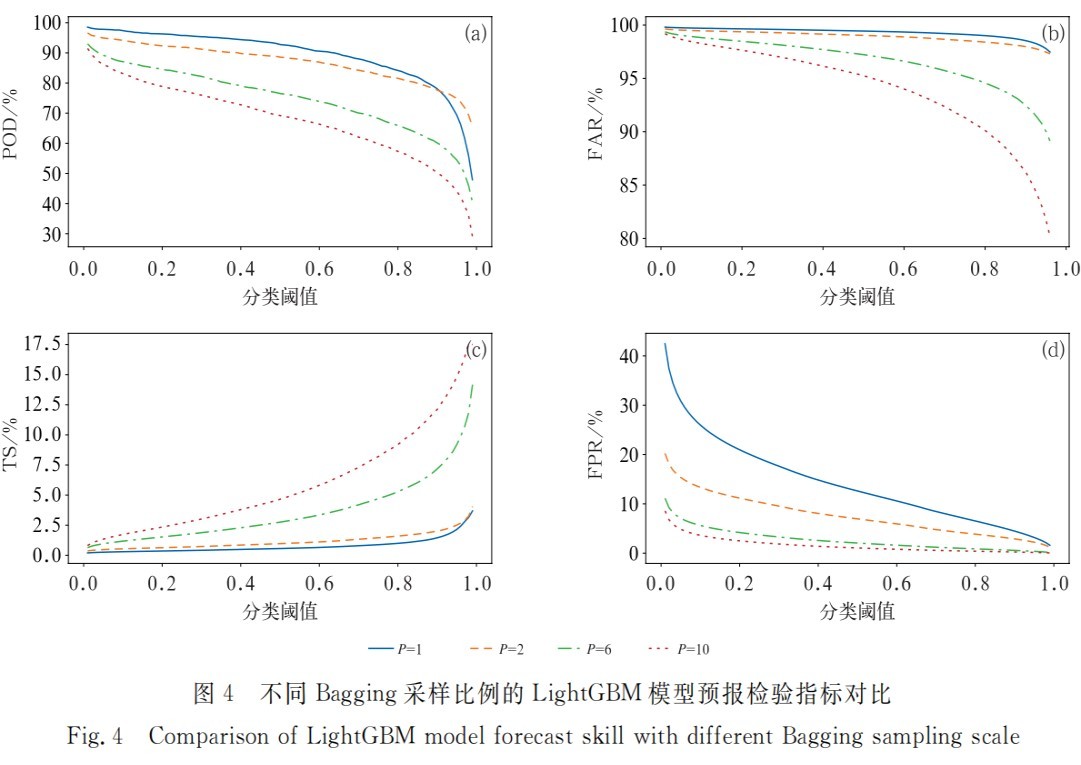