本文已被:浏览 508次 下载 2565次
投稿时间:2020-03-05 修订日期:2020-08-14
投稿时间:2020-03-05 修订日期:2020-08-14
中文摘要: 利用1996—2015年中国的高空探测资料和地面观测数据,挑选发生降水的数十万个样本将其分为降雨和降雪两类事件,抽象为二分类问题,采用深度学习网络技术构建降水相态判识模型,并用2016—2017年的数据进行测试检验,针对2018年1月下旬中国一次大范围雨雪天气过程进行个例检验,在此基础上探讨了深度学习网络在降水相态判识和预报中的应用。主要结论如下:基于深度学习网络判识模型的判识准确率为98.2%,雨、雪的TS评分分别为97.4%和94.4%,相应空报率为1.7% 和2.0%,漏报率为1.0%和3.7%,较传统指标阈值法的判识准确率有较大提高;个例检验显示,基于实况探空数据的模型判识结果与降水相态实况在全国基本保持一致,欧洲中期数值预报中心(ECMWF)的降水相态预报产品和模型的预报结果对全国的降水相态都表现出较好的预报能力,而对雨雪分界线的预报,模型的预报结果较ECMWF总体上更接近实况。测试结果表明,模型较好地提取了雨、雪降水相态的结构特征,深度学习网络在降水相态判识和预报中的应用具有可行性和一定的优势,可为降水相态的客观判识和预报提供重要技术支撑。
中文关键词: 降水相态,深度学习,雨雪分界线,检验
Abstract:This paper applies deep learning method on establishing a model to discriminate the precipitation type. Hundreds of thousands of precipitation samples obtained from sounding and observation data of China from 1996 to 2015 were divided into rain and snow events. The 2016-2017 data were tested, and a case test was conducted on a rain and snow weather process over China in late January 2018. Furthermore, the application of deep learning method to discrimination and forecasting of precipitation type was discussed. The main conclusions are as follows. Discrimination accuracy of the model is 98.2%, which is more improved than the traditional index threshold method. TS scores of rain and snow are 97.4% and 94.4%, false discriminate rates are 1.7% and 2.0%, and omission rates are 1.0% and 3.7%, respectively. The case test denotes that the model discrimination results based on the observation data are basically consistent with the observation data. The ECMWF precipitation type products and the model results also have a good forecast performance for precipitation type over China. Compared with ECMWF, the model forecast for rain and snow separating line is more consistent with observation. The test results show that the discrimination model established in this paper can extract the key features of precipitation type of rain and snow. The application of deep learning method to discrimination and forecasting of precipitation type is feasible and advantageous. Thus, this method could provide important technical support for the objective identification and prediction of precipitation type.
文章编号: 中图分类号: 文献标志码:
基金项目:湖南省气象局第三期业务能力建设项目(NLJS01)、湖南省气象局重点项目(XQKJ17A004)、湖南省水利重点科技项目(XSKJ2018179-07)和湖南省重点领域研发计划项目(2019SK2161)共同资助
引用文本:
黄骄文,蔡荣辉,姚蓉,王胜春,滕志伟,2021.深度学习网络在降水相态判识和预报中的应用[J].气象,47(3):317-326.
HUANG Jiaowen,CAI Ronghui,YAO Rong,WANG Shengchun,TENG Zhiwei,2021.Application of Deep Learning Method to Discrimination and Forecasting of Precipitation Type[J].Meteor Mon,47(3):317-326.
黄骄文,蔡荣辉,姚蓉,王胜春,滕志伟,2021.深度学习网络在降水相态判识和预报中的应用[J].气象,47(3):317-326.
HUANG Jiaowen,CAI Ronghui,YAO Rong,WANG Shengchun,TENG Zhiwei,2021.Application of Deep Learning Method to Discrimination and Forecasting of Precipitation Type[J].Meteor Mon,47(3):317-326.
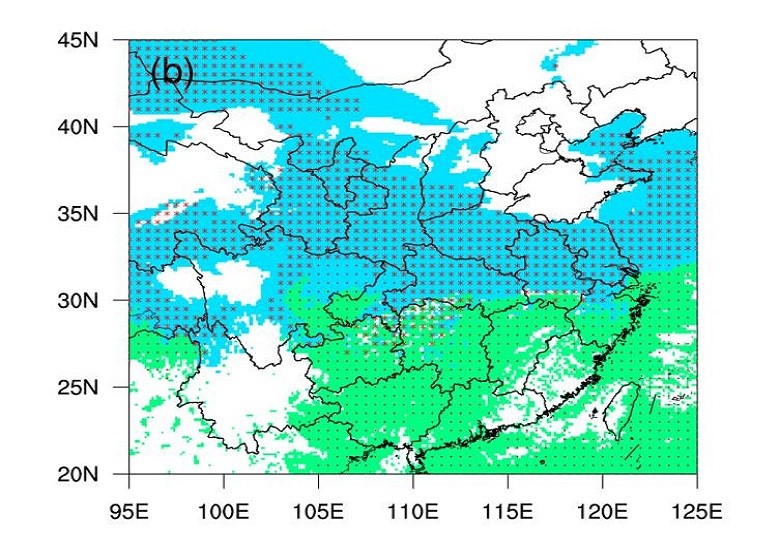