本文已被:浏览 1284次 下载 2227次
投稿时间:2018-07-23 修订日期:2018-12-21
投稿时间:2018-07-23 修订日期:2018-12-21
中文摘要: 通过在湖南南岳高山气象观测站及怀化国家气候基准站设立的外场试验,采集2018年1—3月两站逐分钟积雪天气现象摄像图片,采用卷积神经网络技术对南岳站积雪试验图片进行建模训练,并用南岳站和怀化站的测试图片进行检验,在此基础上探讨了基于深度学习的积雪天气现象图像识别的采集环境布局要求。主要结论如下:南岳站识别的正确率为99.23%,空判率为0.49%,漏判率为0.28%,白天识别结果优于夜间;判识积雪出现的概率在积雪形成初期明显增加,维持期接近99.99%,且十分稳定,融雪时逐步降低;地面积雪形成初期和积雪结束期凝结物较少时偶尔出现漏判,当有雨凇、雾凇及其他背景污染时会出现个别时刻空判现象。怀化站测试结果与南岳站相似,正确率为97.78%,空判率为1.92%,漏判率为0.3%;但概率曲线波动较大,一方面由于怀化站图片没有参与建模训练,另一方面可能与怀化站的摄像头固定不佳、对焦不准、拍摄不清晰有关。测试结果表明:该人工智能判识模型较好地提取了积雪不同发展阶段的关键特征,识别效果良好,并可通过增加气象要素条件和根据判识的前后一致性进一步消除空漏判,可为此类天气现象自动观测提供重要技术支撑。
中文关键词: 地面积雪,人工智能,自动识别
Abstract:Based on field experiments at Nanyue Mountain Meteorological Station and Huaihua National Reference Climatological Station in Hunan Province, the camera images of snow cover weather phenomena were collected minutely from January to March in 2018. The convolution neural network technology is employed for modelling and training using the experimental pictures at Nanyue Station, and the results are examined by the testing pictures of Nanyue Station and Huaihua Station. Furthermore, based on deep learning, the environmental layout requirements of snow cover image identification are discussed. The main conclusions are as follows. Recognition accuracy at Nanyue Station is 99.23%, omission rate is 0.49%, false identification rate is 0.28%, and the recognition result at daytime is better than at night. The accuracy increases to approximately 99.99% with high stability during the formation stage of snow cover, and it decreases with snow melting. There are a few false cases when the snow cover on the ground is in the early stage of snow cover formation and about to melt. There are occasional misjudgment because of background pollution of fog and rime. The test results of Huaihua Station are similar to those for Nanyue Station, with an accuracy of 97.78%, a false identification rate of 1.92% and 0.3% missed, but less stable. This is because of two reasons. First, the data of Huaihua were not used for modelling, and then the cameras were not well fixed leading to bad images. The test results show that the artificial intelligence identification model established in this paper can extract the key features of snow cover in different stages, and the identification result is well. In addition, the false accept and omission can be further eliminated by including the meteorological element conditions and judging the consistency of the snow co ver. This method can provide important technical support for the automatic observation of snow cover weather phenomena.
文章编号: 中图分类号: 文献标志码:
基金项目:中国气象局2018年小型业务建设项目《综合气象观测智能分析判识系统建设》和中国气象局2019年山洪项目《地面气象自动观测智能分析判识系统建设》共同资助
作者 | 单位 |
黄小玉 | 国家气象中心,北京 100081 |
张垚 | 北京我去修科技有限公司,北京 100101 |
叶成志 | 湖南省气象台,长沙 410118 |
潘志祥 | 湖南省气象台,长沙 410118 |
傅承浩 | 湖南省气象台,长沙 410118 |
引用文本:
黄小玉,张垚,叶成志,潘志祥,傅承浩,2019.地面积雪天气现象人工智能观测识别研究[J].气象,45(9):1189-1198.
HUANG Xiaoyu,ZHANG Yao,YE Chengzhi,PAN Zhixiang,FU Chenghao,2019.Research on Artificial Intelligence Observation and Identification of Snow Cover Weather Phenomenon on Surface[J].Meteor Mon,45(9):1189-1198.
黄小玉,张垚,叶成志,潘志祥,傅承浩,2019.地面积雪天气现象人工智能观测识别研究[J].气象,45(9):1189-1198.
HUANG Xiaoyu,ZHANG Yao,YE Chengzhi,PAN Zhixiang,FU Chenghao,2019.Research on Artificial Intelligence Observation and Identification of Snow Cover Weather Phenomenon on Surface[J].Meteor Mon,45(9):1189-1198.
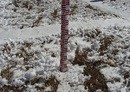