本文已被:浏览 938次 下载 1783次
投稿时间:2018-05-28 修订日期:2018-10-23
投稿时间:2018-05-28 修订日期:2018-10-23
中文摘要: 东亚冬季风强度与中国中东部冬季霾日数的变化在年际尺度上密切相关,这为霾的短期气候预测提供了可能的物理因子。利用NCEP/NCAR再分析资料和安徽省1980—2016年气象观测数据,采用统计分析方法研究安徽省1月霾日数与同期不同东亚季风指数的关系,确定了安徽省不同区域冬季霾的主要季风指数预测因子,建立安徽省冬季霾的月尺度预测模型,并进行了验证。结果表明:(1)1月气候霾日数与6类东亚冬季风指数均呈反相关关系,其中淮河以北、江淮之间两个区东亚大槽强度指数与气候霾日数的相关系数在各项指数中最高,沿江江南为西伯利亚高压强度指数与气候霾日数的相关系数在各项指数中最高。(2)不同分区建立的1月气候霾日数的预测模型均通过了α=0.01的显著性水平检,验证结果表明,霾日数预测等级与实况等级基本一致,各区均未出现预测错误的情况,表明模型具有较好的预测表现。(3)在安徽省冬季霾实际预测业务中,相比NCEP CFS2模式输出的环流预报场,ECMWF SYSTEM4模式输出环流预报场的预测效果更好。
中文关键词: 冬季霾,东亚冬季风,相关关系,预测模型,安徽
Abstract:Interannual variation in the intensity of the East Asian winter monsoon (EAWM) is closely related to that in winter haze days in the middle and eastern China, providing a possible physical factor for short term climate prediction of haze days. Using the NCEP/NCAR reanalysis data and the meteorological observation data of Anhui Province from 1980 to 2016, the statistical analysis method is used to study the relationship between the number of haze days in Anhui Province and six different EAWM indices (EAWMI) in January, and the main EAWMI for winter haze days in different regions of Anhui Province are extracted, and then the monthly predictive model of winter haze days in Anhui Province is established and verified. The results show that: (1) The number of climatic haze days in January is negatively related to the six EAWMI. For both the northern part of the Huaihe River and regions between the Yangtze River and the Huaihe River, the correlation coefficient between the East Asia large trough intensity index and the number of climatic haze days is the highest. For along and to the south of the Yangtze River, the correlation coefficient between the Siberia high intensity index and the number of climatic haze days is the highest. (2) The prediction models for the number of haze days are established in three different regions, and all of them have passed the significance test with α=0.01 level. The verification results show that the predicted haze days are very similar with actual situations, and no predictive errors appear in three regions, which indicates that all the prediction models present a good predictive performance. (3) In the predictive work on winter haze days in Anhui Province, it is better to use the output by the ECMWF SYSTEM4 model than that by NCEP CFS2.
文章编号: 中图分类号: 文献标志码:
基金项目:安徽省自然科学基金(1608085MD84)、国家自然科学基金项目(41601550)、安徽省气象科技发展基金(KM201714)及安徽省重点研究和开发计划(1804a0802215)共同资助
引用文本:
张浩,石春娥,杨元建,2019.基于东亚冬季风指数的安徽省冬季霾预测研究[J].气象,45(3):407-414.
ZHANG Hao,SHI Chune,YANG Yuanjian,2019.Prediction of Winter Haze Days in Anhui Province Based on East Asian Winter Monsoon Index[J].Meteor Mon,45(3):407-414.
张浩,石春娥,杨元建,2019.基于东亚冬季风指数的安徽省冬季霾预测研究[J].气象,45(3):407-414.
ZHANG Hao,SHI Chune,YANG Yuanjian,2019.Prediction of Winter Haze Days in Anhui Province Based on East Asian Winter Monsoon Index[J].Meteor Mon,45(3):407-414.
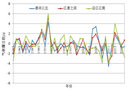